Title: Revolutionizing Object Tracking in Sports with TAPIR: A Breakthrough in Artificial Intelligence
Introduction:
Artificial intelligence (AI) continues to push boundaries and revolutionize various industries. In the realm of sports, AI has the potential to transform the way we analyze and understand the game. One of the most critical aspects of sports analysis is object tracking in video sequences, which provides valuable insights into player movements, ball trajectories, and overall performance. In this blog, we explore how the groundbreaking technology called Tracking Any Point with per-frame Initialization and temporal Refinement (TAPIR) is set to redefine object tracking in sports and its wide-ranging applications.
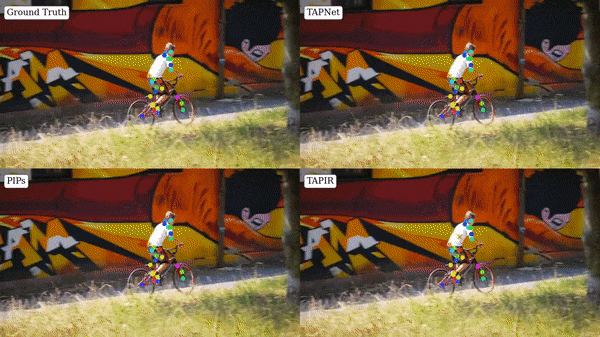
Understanding Object Tracking in Sports:
Object tracking in sports refers to the process of accurately locating and following specific objects, such as players, balls, or equipment, across consecutive frames of a video. By tracking objects' positions, sizes, shapes, and other relevant attributes, we gain invaluable insights that aid in performance analysis, tactical decision-making, and skill evaluation.

Applications of Object Tracking in Sports:
-
Player Analysis: Object tracking allows for comprehensive player analysis by capturing their movements, positions, and interactions. Coaches and analysts can assess player performance, identify strengths and weaknesses, and make data-driven decisions regarding training and strategies.
-
Tactical Analysis: Tracking the movement of players and the ball helps uncover patterns, formations, and tactics employed during a game. Coaches can use this information to optimize team strategies, understand opponents' playing styles, and make informed adjustments during matches.
-
Ball Trajectory Analysis: Tracking the trajectory of the ball provides insights into shooting techniques, passing accuracy, and ball movement patterns. This analysis is particularly useful in sports like soccer, basketball, or tennis, where ball trajectory significantly impacts gameplay.
-
Injury Prevention and Rehabilitation: Object tracking can aid in injury prevention and rehabilitation by monitoring players' movements and identifying potential risk factors. Coaches and medical staff can track workload, detect abnormal movements, and design training programs tailored to individual needs.
The Innovation: Tracking Any Point with per-frame Initialization and temporal Refinement (TAPIR):
Developed by Google DeepMind, University College London, and the University of Oxford, TAPIR represents a groundbreaking advancement in object tracking technology. It addresses the limitations of traditional tracking methods by focusing on the "Track Any Point" (TAP) task. Let's delve into the key components and benefits of TAPIR:
-
Point-Level Correspondence: Unlike traditional methods that rely on bounding boxes or predefined features, TAPIR leverages point-level correspondence. This approach enables more accurate tracking, even in scenarios with occlusions, appearance changes, or non-rigid deformations.
-
Robust Occlusion Handling: TAPIR robustly estimates occlusions and recovers when points reappear. By incorporating search mechanisms and maintaining point correspondence, it effectively handles challenging situations where objects or players are partially or completely occluded.
-
Long-Term Tracking: TAPIR integrates information from multiple consecutive frames, allowing for accurate and robust long-term tracking. It leverages the appearance and motion data across frames to maintain precise object positions and trajectories.
-
Synthetic Data for Training: TAPIR addresses the limited availability of real-world ground truth data by leveraging synthetic data. This allows the model to learn from simulated environments and generalize well to real-world tracking scenarios.
TAPIR Architecture and Implementation:
TAPIR combines the strengths of two existing architectures, TAP-Net and Persistent Independent Particles (PIPs), to achieve highly accurate and continuous object tracking. The model features a fully convolutional architecture that enables efficient mapping onto modern GPU and TPU hardware. TAPIR also estimates its own uncertainty in position estimation, leading to improved benchmark scores and better performance in downstream algorithms.
To train TAPIR, the Kubric MOVi-E dataset is utilized. The model is trained using a cosine learning rate schedule and an Adam optimizer. The inclusion of panning scenarios in the dataset enhances TAPIR's ability to handle real-world videos effectively.
Benefits and Future Implications:
TAPIR's breakthrough in object tracking technology holds immense potential for the sports industry. The accurate and robust tracking of players, balls, and other objects opens up new avenues for performance analysis, tactical planning, and injury prevention. Coaches, analysts, and sports professionals can gain deeper insights into the game, leading to improved strategies, enhanced training programs, and better overall performance.
As AI continues to advance, we can expect further refinement and application of TAPIR-like technologies in sports. Real-time tracking, integration with other AI-driven systems, and enhanced visualization tools are just a few areas where this technology can make a significant impact in the future.
Conclusion:
With the advent of TAPIR, the landscape of object tracking in sports is set to undergo a revolutionary transformation. The ability to accurately track and analyze players, balls, and other objects in videos opens up a world of possibilities for performance analysis, tactical insights, and injury prevention in sports. As we witness the evolution of AI and computer vision technologies, TAPIR stands as a testament to the potential of innovation in shaping the future of sports analysis. Exciting times lie ahead as sports professionals harness the power of AI to gain a competitive edge and push the boundaries of human performance.